Better Project Management
With climate change forcing the pace, many sectors are contemplating major changes in technology and basic infrastructure. The oil- and coal-fired power generators of the last century are giving way to wind farms and solar arrays. Fossil-fueled cars and networks of gas stations may soon be consigned to history. In almost every industry, large capital investments will need to be made, and with them will come big risks.
I’ve researched and consulted on megaprojects for more than 30 years, and I’ve found that two factors play a critical role in determining whether an organization will meet with success or failure: replicable modularity in design and speed in iteration. If a project can be delivered fast and in a modular manner, enabling experimentation and learning along the way, it is likely to succeed. If it is undertaken on a massive scale with one-off, highly integrated components, it is likely to be troubled or fail.
Unfortunately, in conventional business and government megaprojects—such as hydroelectric dams, chemical-processing plants, aircraft, or big-bang enterprise-resource-planning systems—the norm is still to build something monolithic and customized. Such projects must be 100% complete before they can deliver benefits: Even when it’s 95% complete, a nuclear reactor is of no use. Components are typically bespoke, with a high degree of specificity rather than modularity, which limits opportunities for learning and increases the costs of both integration and rework when problems arise. New technologies and customized designs are common, which further hinders speed and modular scale-up. What’s more, the size of megaprojects is typically specified many years before operations are slated to begin. That spells disaster if more capacity is built than is ultimately needed or if demand is greater than expected and additional capacity cannot be added. The Channel rail tunnel between France and the UK, for instance, has a fixed capacity, and because tunnel use is roughly half of what was projected, huge and expensive capacity goes unused. The investment has been a financial calamity.
Cost overruns may not matter if you are a large multinational such as BP or Tesla contemplating a $10 million project. At such firms, coming in at $10 million over budget would make little difference to the bottom line. But when the estimated budget starts at $10 billion, the stakes are much higher, even for governments. Smart organizations, therefore, adopt processes and technologies that lend themselves to modularity and rapid learning and involve less-complicated rework when problems arise.
To entrepreneurs in the tech industry, much of this will sound familiar and logical. But large corporations and governments have yet to internalize these lessons for big-ticket projects. To be sure, many megaprojects—such as bridges or power plants—are unlikely to ever be completely modular, but there is still plenty of scope for choosing technologies that enable rapid scaling and introducing modularity by applying tried-and-true technologies in innovative ways. Let’s begin by considering the factors that enable projects to scale up rapidly.
Why Speed and Modularity Matter
Speed is important to the success of megaprojects because with extended timelines come increased risk and uncertainty. Decades of research by Philip Tetlock, a professor at the Wharton School, have established that people can forecast certain events—such as GDP growth, macroeconomic policies, business cycles, technological advances, and geopolitical conflicts—with some accuracy over periods of up to one year. After that, however, accuracy declines rapidly, and beyond a time horizon of three to five years, it disappears into the mists of randomness.
And Tetlock is probably overly optimistic in that assessment. His findings are based on the work of highly skilled forecasters, and the forecasts he studies are simplified, often framed as yes or no answers to questions such as: “In the next year, will any country withdraw from the euro zone?” or “Will North Korea detonate a nuclear device before the end of this year?” Most real-life forecasts do not have binary answers but instead cover a wide range of possible outcomes. They address questions like: “How many people are likely to die from Covid-19 over the next year?” or “How much is the California high-speed rail system likely to cost?” Binary questions are easier to answer than ones with many possible answers, but the latter are more common in practice.
Entrepreneurs and financiers in Silicon Valley, who compete in winner-take-all markets, have long understood that speed is critical. New ventures in the tech industry place a great emphasis on developing a minimum viable product within their first year and establishing themselves as a market leader within three to five years. LinkedIn cofounder Reid Hoffman calls this process “blitzscaling” and argues that scale-ups, and not start-ups, are what distinguish Silicon Valley from other tech ecosystems.
Speed is only half the equation. Former Alphabet chairman and CEO Eric Schmidt and former Google senior vice president of products Jonathan Rosenberg identify the other half: Ship and iterate. “Create a product, ship it, see how it does, design and implement improvements, and push it back out,” they advise. “The companies that are the fastest at this process will win.”
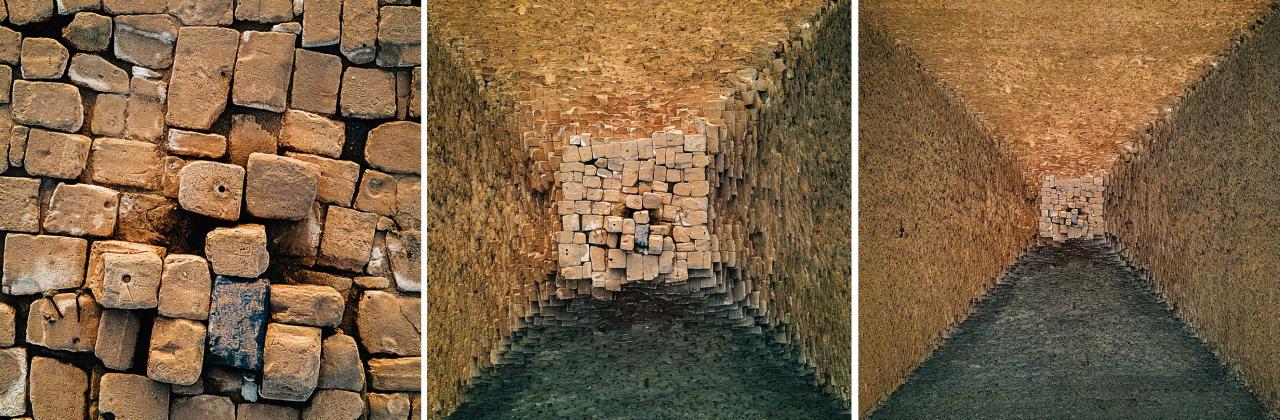
Iteration ensures that the quality of delivery constantly improves as you go along. As Harvard Business School professors emeriti Carliss Baldwin and Kim Clark demonstrated over two decades ago, iteration enables learning by creating a feedback loop in which the experience from delivering one module improves the delivery of the next, repeatedly. Iteration also provides scope for experimentation. Instead of going full-scale immediately, you experiment with a few modules, improve the next ones, and repeat until you master delivery, at which point you go full-scale. It’s easy to see how speed contributes to this process—the faster you iterate, the more you learn and the further you can drive cost down and safety and productivity up.
Humans are inherently good at experimenting and learning, which is why a venture based on modular replicability is more likely to succeed than one that depends on long-range planning and forecasting—something humans are inherently bad at.
Let’s look next at a megaproject that epitomizes a smart scale-up.
Giga Nevada: Scaling Up the Smart Way
Tesla’s Gigafactory 1, also known as Giga Nevada, is a $5 billion high-tech lithium-ion-battery factory under construction east of Reno. The goal of the megaproject is to make electric vehicles and home-power systems more affordable by producing batteries at an unprecedented scale. If completed as planned, Gigafactory 1 will have the biggest footprint in the world, at more than half a million square meters, or 107 football fields.
The building is modular by design. At the outset, Tesla defined a minimum viable production facility, or “block,” that could be operational as soon as it was completed, delivering learning as more blocks were being built. Construction of Gigafactory 1 started in late 2014, and by the third quarter of 2015, the first portion had been finished and was producing the Tesla Powerwall, a home-energy-storage system. In July 2016, Tesla celebrated the grand opening of the factory, with three of 21 blocks completed, amounting to approximately 14% of the expected total size. Mass production of battery cells began in January 2017, just a little over two years after the project broke ground. That pace is much faster than is common in projects of this size, where operations typically start five to seven years after construction begins. In 2014, the projected capacity of Gigafactory 1 was 35 gigawatt-hours a year. That capacity seems to have been achieved even before completion of the factory, indicating that significant learning in construction and manufacturing has taken place.
Tesla reaped two substantial advantages from its emphasis on speed. First, the company lowered the risk of cost overruns, which tend to balloon as schedules drag on. Second, it began generating revenue within a year of deciding to go ahead with the project—much earlier than would have been the case had it used the conventional approach to megaprojects. Both advantages are crucial to fast-growing companies that cannot afford to have funds tied up in slow-moving, risky construction projects.
Unfortunately, traditional megaprojects tend to turn out rather differently.
Monju and the Problem of Negative Learning
Japan’s Monju nuclear power plant, a prototype fast-breeder reactor, was the first of its kind for commercial use. Named after the Buddhist deity of wisdom, it was intended to become the cornerstone of a high-priority national program to reuse and eventually produce nuclear fuel in a country with few energy sources of its own.
The plant was entirely custom designed: Each part and component was created and produced for a unique application and featured cutting-edge technology. Construction got underway in 1986, and initial criticality (that is, a sustained fission chain reaction) was attained eight years later, on schedule, in 1994. Then test operations began, followed by inauguration in August 1995. In December of the same year, a major fire shut down the facility, resulting in a five-year delay, which was considerably extended as further problems were uncovered. Test runs did not begin until 2010, and shortly thereafter, a three-ton machine used for refueling fell into the reactor vessel. It took nearly a year to retrieve the machine.
Following further problems and the discovery of serious maintenance flaws, in May 2013 Monju was ordered to suspend its preparations for restarting the reactor for commercial use. The Nuclear Regulation Authority declared the operator of Monju unqualified to operate the reactor, and in December 2016, the government closed the plant permanently.
After more than 30 years and $12 billion in expenditures, Monju is said to have generated electricity for all of one hour during its 22-year lifetime. Decommissioning is expected to take another 30 years, until 2047, at a further cost of $3.4 billion. If previous experience is anything to go by, those numbers are optimistic, with additional delays and cost overruns a near certainty. At a minimum, Monju will end up a 60-year, $15 billion venture with zero or negative benefits. Monju is not alone—it is merely one of the most obvious examples.
The contrast with Tesla could not be starker. There was nothing in Monju’s design that compared with the replicable production modules at Gigafactory 1, where learning was continual and scaling got better and better and faster and faster. At Monju, everything was done just once, with extreme complexity. That created a phenomenon that operations experts call negative learning, a dynamic in which learning slows rather than accelerates progress. The more the Monju team learned, the more obstacles and additional necessary work it identified.
Like Monju, many megaprojects are difficult to break down into replicable units that can be rapidly iterated to deliver learning and improvement. As soon as you dig a hole in the ground, for example, things seem to become unpredictable, bespoke, and slow. But difficult does not mean impossible. In almost any project, large parts of the work can be made replicable, giving even the least-scalable projects some room for turning negative learning positive. The choice is not an either/or: scalable or not scalable. It is a matter of degree: getting as much scalability as you can into any project, including the least likely ones.
Let’s look at an example.
Madrid’s Modular Metro
Manuel Melis Maynar understands the importance of scalability. An experienced civil engineer and the president of Madrid Metro, he was responsible for one of the largest and fastest subway expansions in history. Subway construction is generally seen as custom and slow by nature. It can easily take 10 years from the decision to invest in a new line until trains start running, as was the case with Copenhagen’s recent City Circle Line. And that’s if you don’t encounter problems, in which case you’re looking at 15 to 20 years, as happened with London’s Victoria line. Melis figured there had to be a better way, and he found it.
Begun in 1995, the Madrid subway extension was completed in two stages of just four years each (1995 to 1999: 56 kilometers of rail, 37 stations; 1999 to 2003: 75 kilometers, 39 stations), thanks to Melis’s radical approach to tunneling and station building. In project management terms, it offers a stark contrast to the experience of the Eurotunnel, which has cost its investors dearly. Melis’s success was the result of applying three basic rules to the design and management of the project.
No monuments.
Melis decided that no signature architecture would be used in the stations, although such embellishment is common, sometimes with each station built as a separate monument. (Think Stockholm, Moscow, Naples, and London’s Jubilee line.) Signature architecture is notorious for delays and cost overruns, Melis knew, so why invite trouble? His stations would each follow the same modular design and use proven cut-and-cover construction methods, allowing replication and learning from station to station as the metro expanded.
No new technology.
The project would eschew new construction techniques, designs, and train cars. Again, this mindset goes against the grain of most subway planners, who often pride themselves on delivering the latest in signaling systems, driverless trains, and so on. Melis was keenly aware that new product development is one of the riskiest things any organization can take on, including his own. He wanted none of it. He cared only for what worked and could be done fast, cheaply, safely, and at a high level of quality. He took existing, tried-and-tested products and processes and combined them in new ways. Does that sound familiar? It should. It’s the way Apple innovates, with huge success.
Speed.
Melis understood that time is like a window. The bigger it is, the more bad stuff can fly through it, including unpredictable catastrophic events, or so-called black swans. He thought long and hard about how to make his window radically smaller by organizing tunneling work for speed. Traditionally, cities building a metro would bring in one or two tunnel-boring machines to do the job. Melis instead calculated the optimal length of tunnel that one boring machine and team could deliver—typically three to six kilometers in 200 to 400 days—divided the total length of tunnel he needed by that amount, and then hired the number of machines and teams required to meet the schedule. At times, he used up to six machines at once, completely unheard of when he first did it. His module unit was the optimal length of tunnel for one machine, and like the station modules, the tunnel modules were replicated over and over, facilitating positive learning.
As an unforeseen benefit, the tunnel-boring teams began to compete with one another, accelerating the pace further. They’d meet in Madrid’s tapas bars at night and compare notes on daily progress, making sure their team was ahead, transferring learning in the process. And by having many machines and teams operating at the same time, Melis could also systematically study which performed best and hire them the next time around. More positive learning. A feedback system was set up to avoid time-consuming disputes with community groups, and Melis persuaded them to accept tunneling 24/7, instead of the usual daytime and weekday working hours, by asking openly if they preferred a three-year or an eight-year tunnel-construction period.
No monuments, no innovation, modular, and fast. Sounds like a recipe for boring, low-quality design, right? But go to Madrid and you will find large, functional, airy stations and trains—nothing like the dark, cramped catacombs of London and New York. Melis’s metro is a workhorse, with no fancy technology to disrupt operations. It transports millions of passengers, day in and day out, year after year, exactly as it is supposed to do. Melis achieved this at half the cost and twice the speed of industry averages—something most thought impossible.
A Wiser Path
The contrasting experiences of the major projects presented here suggest that in embarking on big ventures, companies and governments need to carefully choose and wisely invest in technologies that lend themselves to smart scaling.
Consider again the energy industry. In order to survive, it has to break the current vicious circle of negative learning and crack the code of fast, replicable scale-up. Small modular reactors (SMRs)—nuclear power plants costing an estimated $1 billion each—aim to do just that. Financed by Bill Gates and Warren Buffett, the proposed construction of an SMR in Wyoming may be a first step in that direction. But with an estimated timeline of seven years for the project, it is still very slow. With the climate crisis looming, we don’t have time to wait.
In terms of scalability, a superior alternative is wind. Turbines are inherently modular and replicable—and are thus ideal candidates for smart scale-up. Initially they were constructed on-site, but the nascent industry quickly learned that this was inefficient and shifted to manufacturing them indoors, using industrial processes and logistics that could be effectively controlled and optimized. The UK’s London array was the largest offshore wind farm in the world when it was completed in 2013, costing $3 billion in 2012 prices. The project broke ground in March 2011, electricity production began in October 2012, and all turbines were fully operational by April 2013, just two years and a month after construction started. And by today’s standards, less than a decade later, that’s no longer particularly fast. In 2018, the Walney Wind Farm extension, which is off the coast of England and has 87 turbines, was built in less than a year.
Energy is not the only sector that is starting to move away from the traditional megaproject. Consider the space industry. NASA typically takes a decade to plan and another decade to build its complicated designs. Its missions are too big to fail and too slow to start over when they do. The longer the time, the higher the risk of ultimate failure, with little opportunity to learn along the way. But a new generation of space entrepreneurs (Elon Musk among them) are dramatically lowering costs and delivery times by relying on the use (and reuse) of standard, industrially manufactured building blocks.
Take the case of Will Marshall, who began his career as a young engineer working at NASA’s Jet Propulsion Laboratory. Eventually, he got tired of the slowness and waste of Big Space and decided to do things differently. Along with two other NASA alumni, he founded Planet Labs and built a satellite called Dove in his garage in Cupertino, California.
With a weight of 10 pounds, a build time of a few months, and a cost under $1 million (including launch and operations), Dove satellites are radically smaller, faster, and cheaper to build than anything at NASA—but they are equally well engineered and more agile. Each satellite is made up of three CubeSat modules, which are themselves made up of multiples of 10x10x10 cm modules—what Marshall calls Legos. The CubeSats use commercial off-the-shelf components for their electronics and structure, like those mass-produced for cell phones and recreational drones, keeping cost and delivery times low. During the 2010s, Planet Labs launched several hundred satellites—the largest constellation ever put into orbit—that provide up-to-date information for climate monitoring, farming, disaster response, and urban planning.
Planet Labs lost 26 Dove satellites in 2014. They were sitting on a big rocket that exploded on the launchpad. Stacked against his nine successful launches at the time, the loss hardly affected the business, however. The lost satellites were quickly replaced, and the new ones were put into orbit. Marshall’s modular approach means that every mission is cheap enough to fail and fast enough to replicate in the event of failure, with lessons immediately applied in the next iteration.
. . .
My advice to anyone planning a big project is to follow the examples of Tesla, Planet Labs, Madrid, and wind farms. Where you can, pick basic technologies that lend themselves to modularity and replicability. Where that’s difficult, try to apply regular, tested technologies in innovative, modular ways so that you can learn as you go, driving down cost and accelerating speed with every iteration. If this approach can be effective with something as difficult and necessarily bespoke as digging a subway under a city, it can work for most any project. The possibilities are as rich as your imagination.
Thế giới bản tin | Vina Aspire News
Nguồn : https://hbr.org/2021/11/better-project-management